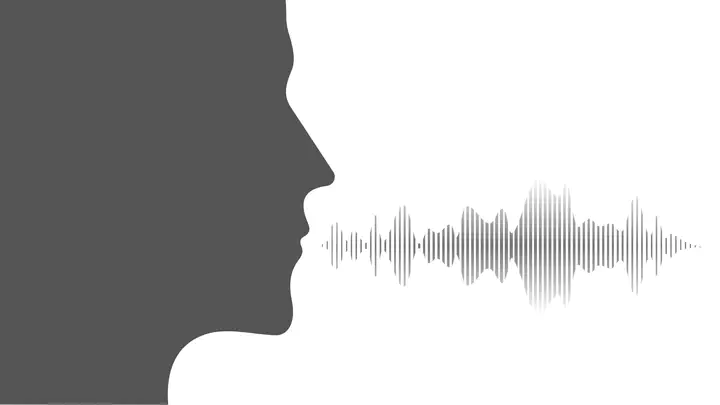
Idiopathic Parkinson’s disease (IDP) is the second most common neurodegenerative disorder worldwide. Due to the non-existence of a cure, current treatments only focus on improving the quality of life by trying to slow the progression of the disease. Therefore, patients require constant monitoring and continuous visits to clinics. Because of this, it is necessary to identify biological markers that allow not only early diagnosis of the disease but also telemonitoring of patients. Different parameters of vocal signals can be used as potential markers for monitoring Parkinson’s disease. In this article, we present the results of the application of a Machine Learning model for the selection of relevant voice parameters to monitor a patient with IPD. We used a database containing 195 voice records from healthy individuals and IPD patients. The classification model that obtained the best result was k-nearest neighbors, with an accuracy of 97.8%. We also identified the HNR, RPDE, and DFA attributes as potential voice parameters for patient diagnosis and monitoring.